Title: How generative AI models such as ChatGPT can be (mis)used in SPC practice, education, and research? An exploratory study
Authors & Year: Megahed, F.M., Chen, Y.J., Ferris, J.A., Knoth, S., and Jones-Farmer, L.A. (2023)
Journal: Quality Engineering [DOI:10.1080/08982112.2023.2206479]
Review Prepared by David Han
Statistical Process Control (SPC) is a well-established statistical method used to monitor and control processes, ensuring they operate at optimal levels. With a long history of application in manufacturing and other industries, SPC helps detect variability and maintain consistent quality. Tools like control charts play a central role in identifying process shifts or trends, allowing timely interventions to prevent serious defects. Megahed, et al. (2023) explores how generative AI, particularly ChatGPT, can enhance the efficiency of SPC tasks by automating code generation, documentation, and educational support. While AI shows promise for routine tasks, the study also highlights its limitations in handling more complex challenges. For instance, ChatGPT’s misunderstanding of core SPC concepts underscores the importance of human oversight to ensure accuracy and prevent errors.
Generative AI’s Role in SPC
As illustrated in Figures 1 and 2, generative AI has seen remarkable growth over recent years. Figure 1 maps out significant milestones in AI’s development, including models like GPT-3 and its rapid adoption across industries. Figure 2 gives insight into the training process of ChatGPT, explaining how it generates human-like text based on vast amounts of data. Together, these provide context for understanding the capabilities of AI in SPC and its growing role in helping professionals complete tasks more efficiently. In the domain of SPC, ChatGPT can handle structured tasks such as generating code or explaining common concepts, with a degree of efficiency that can enhance productivity. For instance, the AI was able to generate R and Python code for control charts – one of the main tools used in SPC for monitoring process stability. ChatGPT successfully translated coding tasks between programming languages, allowing SPC professionals to quickly adapt their processes without deep technical expertise in multiple languages. Additionally, ChatGPT has proven useful in offering frameworks for structuring educational content and brainstorming research ideas, positioning itself as a valuable tool for organizing and simplifying complex tasks. However, the study also revealed ChatGPT’s limitations, especially when tasks require nuanced understanding or involve complex, multi-step processes. For example, while the AI can generate functional code for well-defined tasks, it struggles with more intricate or less familiar scenarios. As illustrated in Figure 3, ChatGPT provided an incorrect description of SPC phases, claiming that there were five phases instead of the typical two phases (Phase 1 and Phase 2) used in SPC process monitoring. The detailed but incorrect explanation it generated in Figure 4 shows the risks of relying on AI in scenarios where accuracy is critical.
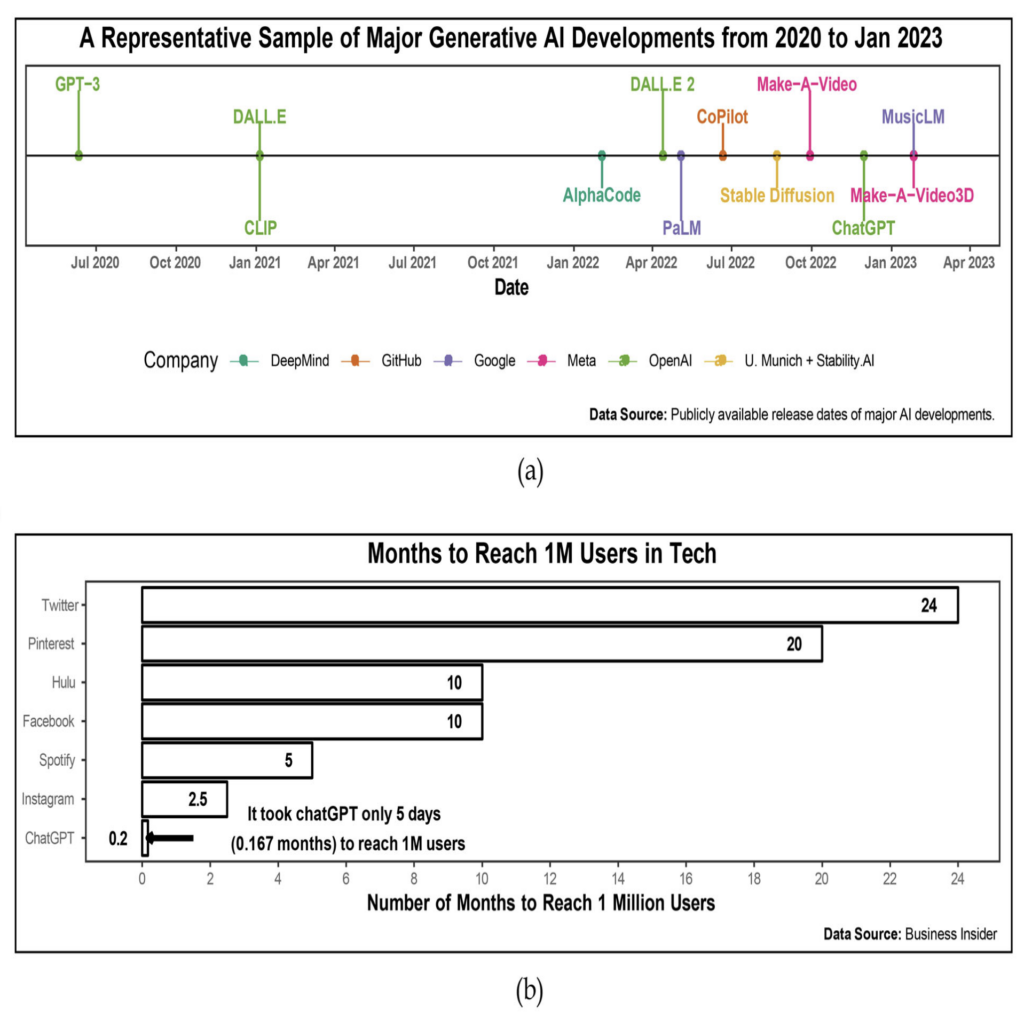
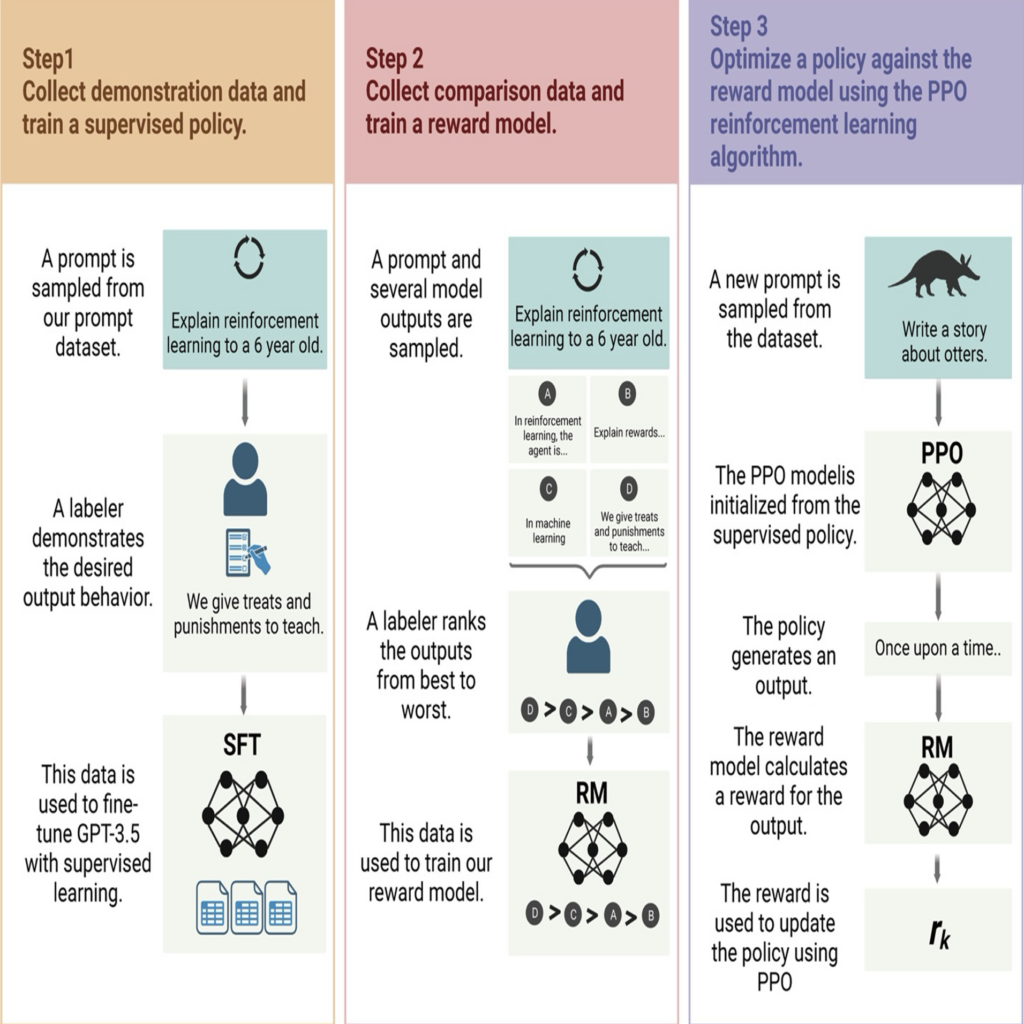
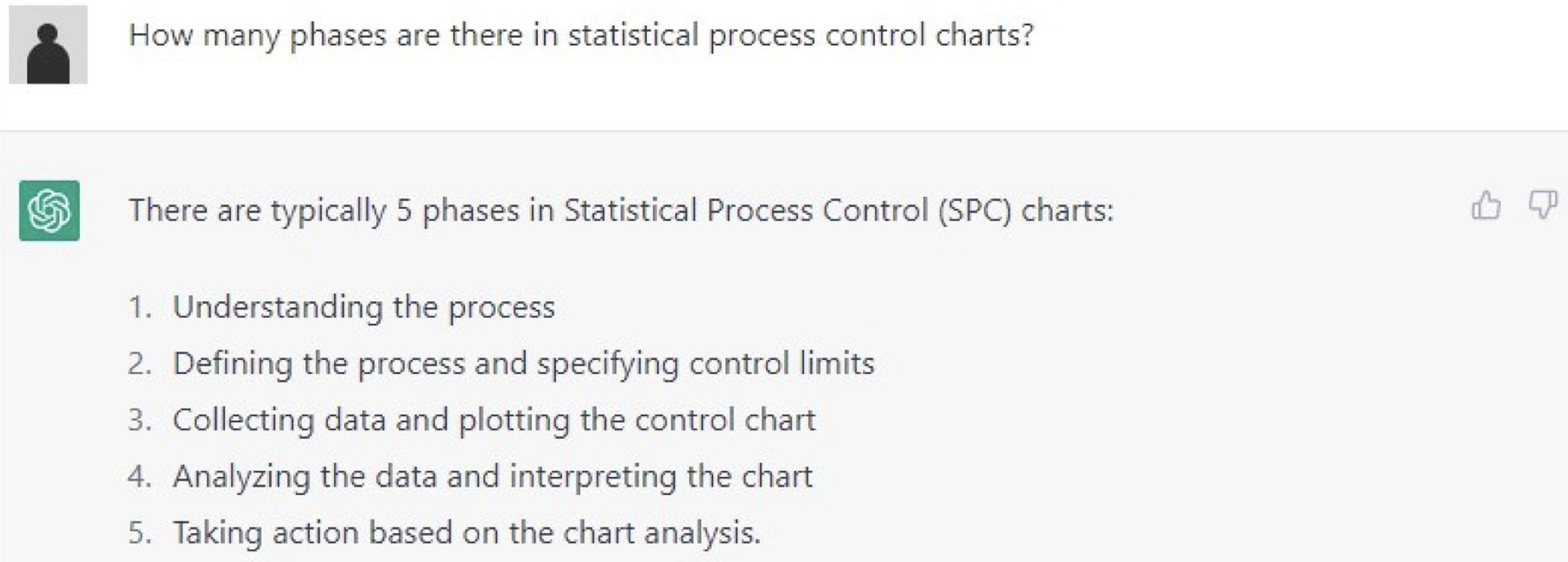
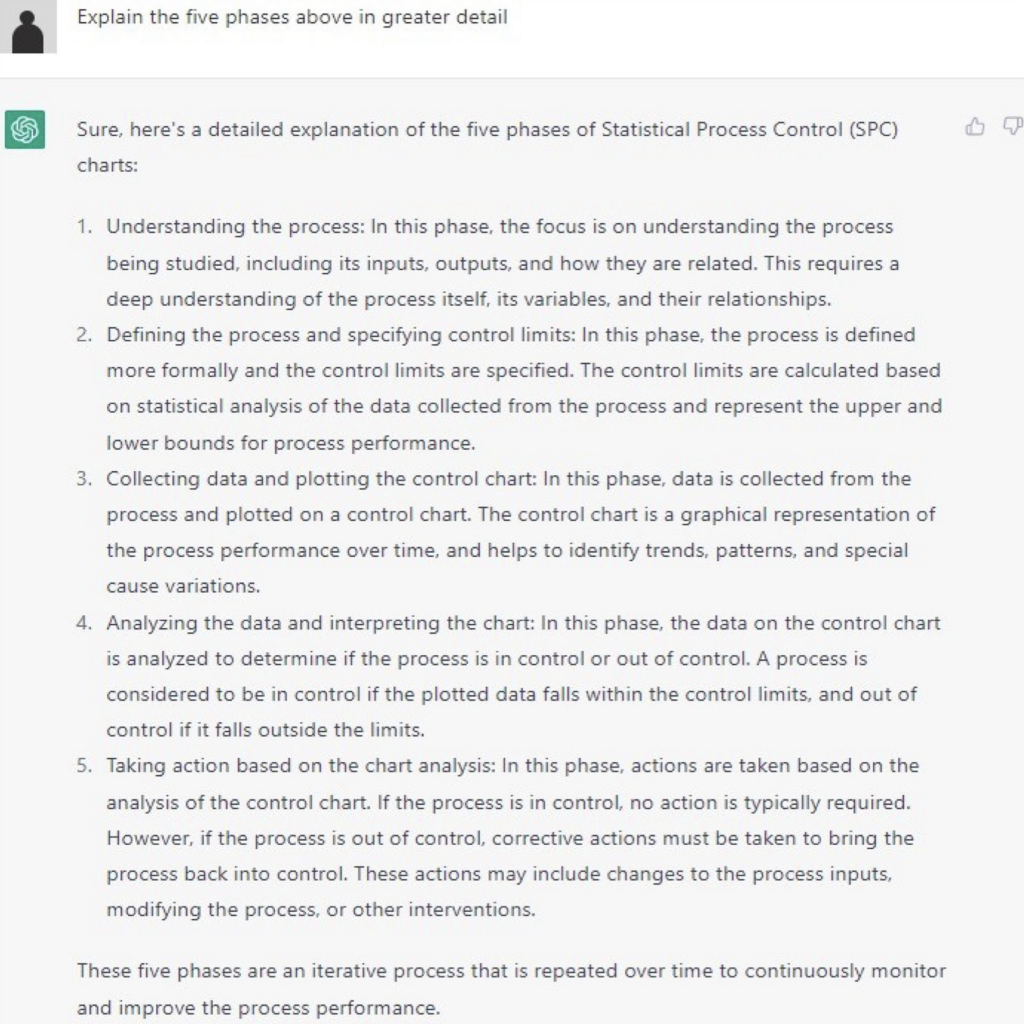
Benefits & Limitations in SPC Practice & Education
In practical settings, ChatGPT offers clear benefits, especially when used for routine or well-structured tasks. It can save time by automating parts of the coding process, translating code between languages, and even summarizing main SPC concepts for reports or documentation. For example, ChatGPT was able to create code for generating X-bar control charts in both R and Python. While the Python code worked seamlessly, the R code required some manual adjustments due to errors in the AI’s assumptions. These errors, though minor, highlight that even in routine tasks, the AI’s output should be carefully reviewed to ensure functionality. ChatGPT’s ability to generate templates and educational materials also makes it useful in SPC education. It can quickly generate course syllabi, lecture topics, and simple explanations of SPC concepts, giving educators a helpful starting point. In one instance, ChatGPT was tasked with creating a course syllabus for an undergraduate SPC class and successfully provided a detailed week-by-week outline that educators could refine for their specific needs. However, the AI’s explanations sometimes lacked depth or precision as it incorrectly explained the difference between Phase 1 and Phase 2 control charts. These kinds of inaccuracies can be particularly problematic in educational settings, where students may not yet have the expertise to recognize incorrect information. Thus, the authors emphasized the need for educators and professionals to verify AI-generated content. While ChatGPT can be a helpful tool for providing quick examples or explanations, its tendency to occasionally produce plausible but incorrect responses makes it unreliable for teaching complex topics without oversight. ChatGPT’s explanation of main SPC terms, though confident, was incomplete or wrong, and this could mislead learners or new practitioners if left unchecked.
Challenges in SPC Research & Knowledge Generation
In research, ChatGPT shows potential for idea generation and knowledge organization but falls short of delivering groundbreaking insights. The study tested ChatGPT’s ability to assist with more complex research tasks such as identifying open research questions or explaining advanced concepts like zero-state ARL (average run length). The AI was able to provide a reasonable list of open issues in SPC research but primarily drew from existing knowledge, offering little in terms of innovative or novel contributions. The limitations of AI-generated knowledge become even more apparent when the task requires deep understanding or precision. For instance, Figure 3 captures an example of how ChatGPT misunderstood the structure of SPC control chart phases, creating an incorrect explanation based on false assumptions. Such errors demonstrate that while ChatGPT can be a useful brainstorming tool, it is not yet sophisticated enough to replace expert judgment in research. Despite these limitations, ChatGPT can still support researchers in more routine or administrative tasks such as generating initial frameworks for research proposals or summarizing findings. However, researchers must treat AI outputs as drafts that require careful review and refinement. Inaccuracies like those seen in Figures 3 and 4 underscore the risks of unverified use, where the AI may confidently present wrong information that seems correct on the surface (viz., AI hallucinations and/or systemic bias).
Conclusion & Future Prospects
The article presents a nuanced view of ChatGPT’s role in SPC, highlighting both its potential and its shortcomings. For tasks that are straightforward, such as generating code or structuring educational materials, ChatGPT can be highly effective. However, for more technical or complex tasks, its limitations are evident as seen in its misunderstanding of SPC concepts in Figures 3 and 4. Human oversight is essential to ensure that AI-generated content is accurate, especially in fields like SPC, where precision and reliability are critical. Looking forward, the role of generative AI in SPC will likely expand as these tools become more refined. As AI models evolve, they may become more reliable for use in complex scenarios, potentially reducing the need for manual review in certain tasks. However, for now, ChatGPT and similar models serve best as complementary tools to human expertise rather than replacements. The authors also stress that experts should remain involved in validating and refining AI-generated content to avoid errors that could have significant consequences in practice and education.